There is plenty of material online for learning machine learning. I like to share some links in this post to what I know. Feel free to add comments with other material.
Stanford: Deep Learning for Natural Language Processing
Natural language processing (NLP) is one of the most important technologies of the information age. Understanding complex language utterances is also a crucial part of artificial intelligence. Applications of NLP are everywhere because people communicate most everything in language: web search, advertisement, emails, customer service, language translation, radiology reports, etc. There are a large variety of underlying tasks and machine learning models powering NLP applications. Recently, deep learning approaches have obtained very high performance across many different NLP tasks. These models can often be trained with a single end-to-end model and do not require traditional, task-specific feature engineering. In this spring quarter course students will learn to implement, train, debug, visualize and invent their own neural network models. The course provides a deep excursion into cutting-edge research in deep learning applied to NLP. The final project will involve training a complex recurrent neural network and applying it to a large scale NLP problem. On the model side we will cover word vector representations, window-based neural networks, recurrent neural networks, long-short-term-memory models, recursive neural networks, convolutional neural networks as well as some very novel models involving a memory component. Through lectures and programming assignments students will learn the necessary engineering tricks for making neural networks work on practical problems.
They provide YouTube videos and slides. The videos are simply recordings of the lecture, but in good quality.
Udacity: Intro to Machine Learning
Machine Learning is a first-class ticket to the most exciting careers in data analysis today. As data sources proliferate along with the computing power to process them, going straight to the data is one of the most straightforward ways to quickly gain insights and make predictions.
Machine learning brings together computer science and statistics to harness that predictive power. It’s a must-have skill for all aspiring data analysts and data scientists, or anyone else who wants to wrestle all that raw data into refined trends and predictions.
This is a class that will teach you the end-to-end process of investigating data through a machine learning lens. It will teach you how to extract and identify useful features that best represent your data, a few of the most important machine learning algorithms, and how to evaluate the performance of your machine learning algorithms.
Link: udacity.com/course/ud120
- Lessons 1-4: Supervised Classification
- Lesson 5: Datasets and Questions
- Lesson 6 and 7: Regressions and Outliers
- Lesson 8: Unsupervised Learning
- Lessons 9-12: Features, Features, Features
- Lessons 13-14: Validation and Evaluation
- Lesson 15: Wrapping it all Up
Udacity: Intro to Artificial Intelligence
Artificial Intelligence (AI) is a field that has a long history but is still constantly and actively growing and changing. In this course, you’ll learn the basics of modern AI as well as some of the representative applications of AI. Along the way, we also hope to excite you about the numerous applications and huge possibilities in the field of AI, which continues to expand human capability beyond our imagination.
Link: udacity.com/course/cs271
- Part I: Fundamentals of AI
- Overview of AI
- Statistics, Uncertainty, and Bayes networks
- Machine Learning
- Logic and Planning
- Markov Decision Processes and Reinforcement Learning
- Hidden Markov Models and Filters
- Adversarial and Advanced Planning
- Part II: Applications of AI
- Image Processing and Computer Vision
- Robotics and robot motion planning
- Natural Language Processing and Information Retrieval
Udacity: Artificial Intelligence for Robotics
Learn how to program all the major systems of a robotic car from the leader of Google and Stanford's autonomous driving teams. This class will teach you basic methods in Artificial Intelligence, including: probabilistic inference, planning and search, localization, tracking and control, all with a focus on robotics. Extensive programming examples and assignments will apply these methods in the context of building self-driving cars.
Link: udacity.com/course/cs373
- Lesson 1: Localization
- Lesson 2: Kalman Filters
- Lesson 3: Particle Filters
- Lesson 4: Search
- Lesson 5: PID Control
- Lesson 6: SLAM (Simultaneous Localization and Mapping)
Side notes
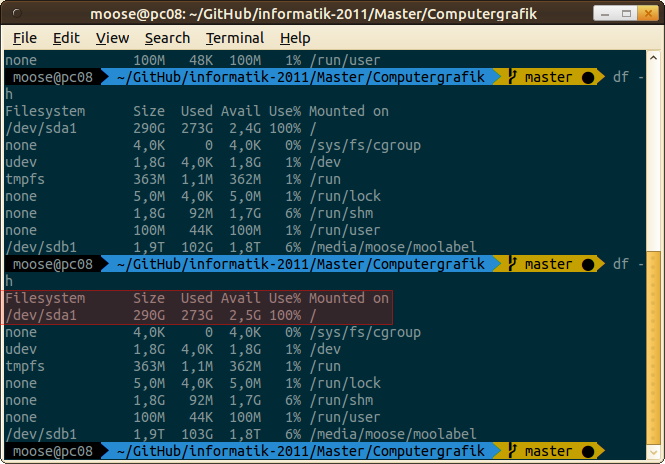
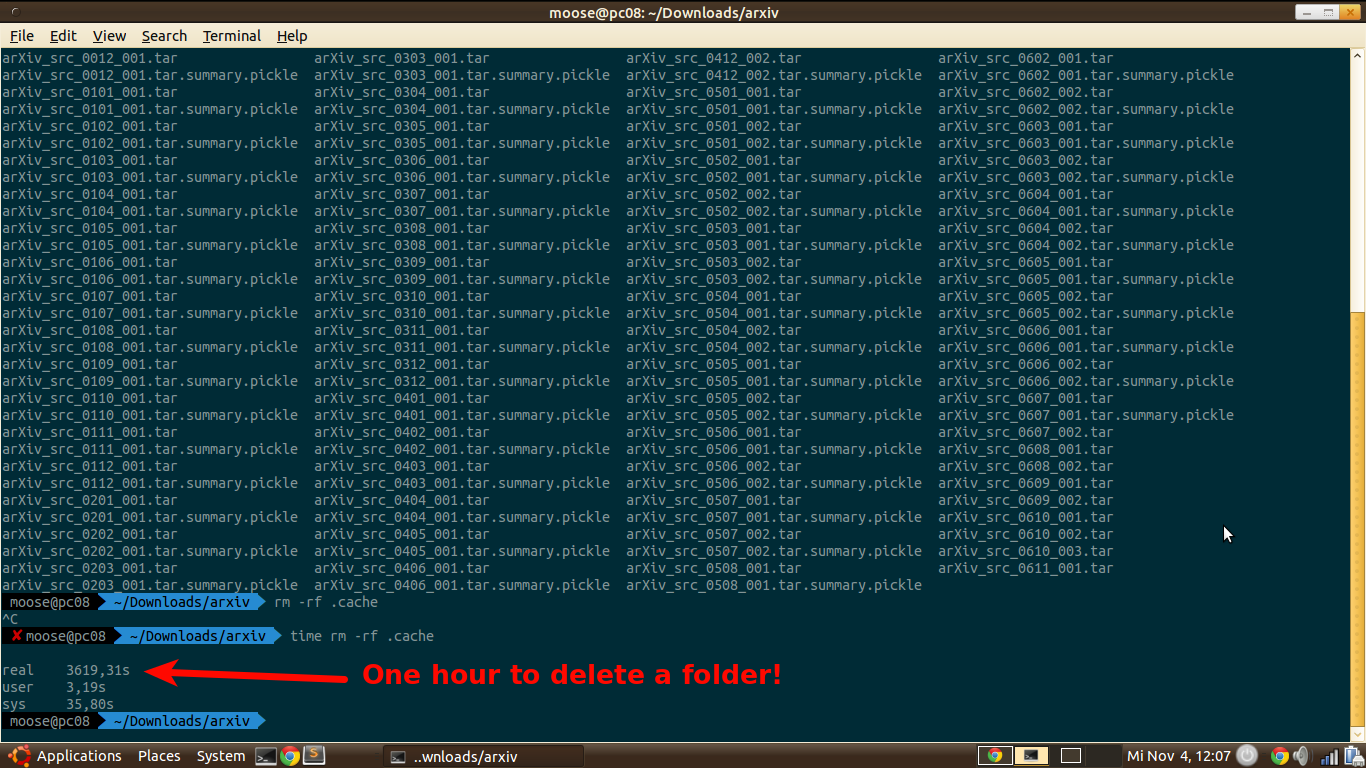
More links
- Learning Deep Learning: A list of deep learning books, papers and websites.
- Deep Learning, Feature Learning
- Deep Learning Master Class
- Tutorial on Deep Learning for Vision
- Neural Network Debugging
- Machine Learning Glossary