This post is about simple implementations of matrix multiplications. The goal of this post is to find out how easy it is to implement a matrix multiplication in Python, Java and C++. Additionally, I want to get to know how good these solutions are.
The second post will be an implementation of the Strassen algorithm for matrix multiplication. Strassen algorithm does matrix multiplication in $\cal O(n^{log_2(7)+o(1)}) \approx \cal O(n^{2.807})$ instead of $\cal O(n^3)$. I am quite sure this will outperform almost every other change. See Part II: The Strassen algorithm in Python, Java and C++.
The third post will be about parallel programming. I have two cores and I want to see if it will be significantly faster if I use both of them.
The implementations ¶
I will post all scripts for this test and I've added a GIT repository, so feel free to test it on your machine. I am also happy if you post some of your solutions with running times ☺ I am quite sure that my Java and C++ code can be written much better. If you know how, please leave a comment. If you know other languages, you could create a script for these. I focus on Python, Java and C++ as they are very often used.
I have implemented these three types of algorithms for this post:
- ijk-algorithm: This is a simple, straight forward implementation of a matrix multiplication. I've used the definition of matrix multiplication. I didn't use multiple threads.
- ikj-algorithm: just like the ijk-algorithm, but I've switched two of the three the for-loops.
- Library-functions: I always prefer libraries over self-implemented solutions. I think they are faster than anything I could come up with in a reasonable amount of time.
If you post a solution, please consider these restrictions:
- Input: The input file should get passed with the parameter
-i
, e.g.:python -i 2000.in
orjava Shell -i 2000.in
- The standard value for the command line parameter -i should be "2000.in" (a $2000 \times 2000$ matrix)
- The user should not have to give the size of the matrix!
- The two square-matrices that should get multiplied are ...
- ... read from a text-file.
- ... represented like this:
- Every line of one matrix is one line in the text-file.
- Newlines are only "\n".
- Every number is separated by "\t".
- The both matrices are separated by one newline.
- Output: The result has to get printed to standard output.
- The result has to be formatted like the input (tabs for separation of number, \n for marks a new line)
The Tests ¶
I will check the speed of a multiplication of two big matrices following for Python, Java and C++ for all algorithms like this:
$ time python scriptABC.py -i ../2000.in > result.txt
$ diff result.txt bigMatrix.out
The bigMatrix.out
was produced by the Python ijk-implementation. I make the diff to test if the result is correct.
The Setting ¶
I created two "random" matrices $A, B \in \mathbb{N}^{2000 \times 2000}$ with this script. The file that was created needs about 29.7 MB and is also in the GIT-Hub repository. But you can also create the matrices with this script:
#!/usr/bin/env python
# -*- coding: utf-8 -*-
import random
random.seed(1234)
def createRandomMatrix(n):
maxVal = 1000 # I don't want to get Java / C++ into trouble
matrix = []
for i in range(n):
matrix.append([random.randint(0, maxVal) for el in range(n)])
return matrix
def saveMatrix(matrixA, matrixB, filename):
f = open(filename, "w")
for i, matrix in enumerate([matrixA, matrixB]):
if i != 0:
f.write("\n")
for line in matrix:
f.write("\t".join(map(str, line)) + "\n")
n = 3
matrixA = createRandomMatrix(n)
matrixB = createRandomMatrix(n)
saveMatrix(matrixA, matrixB, "2000.in")
All scripts are tested on my computer:
Acer TravelMate 5735Z | |
CPU | 2x Pentium(R) Dual-Core CPU T4500 @2.30GHz |
RAM | 4 GB |
Video Card | Intel GMA 4500MHD |
System | Ubuntu 10.10.04 LTS |
Python ¶
I've used Python 2.6.5.
ijk-algorithm ¶
#!/usr/bin/env python
# -*- coding: utf-8 -*-
from optparse import OptionParser
parser = OptionParser()
parser.add_option(
"-i",
dest="filename",
default="2000.in",
help="input file with two matrices",
metavar="FILE",
)
(options, args) = parser.parse_args()
def read(filename):
lines = open(filename, "r").read().splitlines()
A = []
B = []
matrix = A
for line in lines:
if line != "":
matrix.append(map(int, line.split("\t")))
else:
matrix = B
return A, B
def printMatrix(matrix):
for line in matrix:
print("\t".join(map(str, line)))
def standardMatrixProduct(A, B):
n = len(A)
C = [[0 for i in range(n)] for j in range(n)]
for i in range(n):
for j in range(n):
for k in range(n):
C[i][j] += A[i][k] * B[k][j]
return C
A, B = read(options.filename)
C = standardMatrixProduct(A, B)
printMatrix(C)
real 56m49.266s
user 56m30.524s
sys 0m2.980s
ikj-algorithm ¶
def ikjMatrixProduct(A, B):
n = len(A)
C = [[0 for i in range(n)] for j in range(n)]
for i in range(n):
for k in range(n):
for j in range(n):
C[i][j] += A[i][k] * B[k][j]
return C
real 44m36.507s
user 44m13.458s
sys 0m2.000s
Psyco ikj-algorithm ¶
Psyco is a just in time compiler, which makes my scripts MUCH faster. It is very simple to use. Add these two lines at the top of the ikj-script:
import psyco
psyco.full()
real 6m14.820s
user 6m12.959s
sys 0m0.620s
Amazing, isn't it?
Libraries ¶
NumPy ¶
NumPy-Version: 1.3.0 (Current version is 1.6.2, see Wiki)
#!/usr/bin/env python
# -*- coding: utf-8 -*-
import numpy
from optparse import OptionParser
parser = OptionParser()
parser.add_option(
"-i",
dest="filename",
default="2000.in",
help="input file with two matrices",
metavar="FILE",
)
(options, args) = parser.parse_args()
def read(filename):
lines = open(filename, "r").read().splitlines()
A = []
B = []
matrix = A
for line in lines:
if line != "":
matrix.append(map(int, line.split("\t")))
else:
matrix = B
return A, B
def printMatrix(matrix):
matrix = numpy.array(matrix)
for line in matrix:
print("\t".join(map(str, line)))
A, B = read(options.filename)
A = numpy.matrix(A)
B = numpy.matrix(B)
C = A * B # easy and intuitive, isn't it?
printMatrix(C)
real 1m38.425s
user 1m36.066s
sys 0m0.520s
SciPy ¶
You might need to install python-scitools
.
#!/usr/bin/env python
# -*- coding: utf-8 -*-
import numpy
import scipy
from optparse import OptionParser
parser = OptionParser()
parser.add_option(
"-i",
dest="filename",
default="2000.in",
help="input file with two matrices",
metavar="FILE",
)
(options, args) = parser.parse_args()
def read(filename):
lines = open(filename, "r").read().splitlines()
A = []
B = []
matrix = A
for line in lines:
if line != "":
matrix.append(map(int, line.split("\t")))
else:
matrix = B
return A, B
def printMatrix(matrix):
matrix = numpy.array(matrix)
for line in matrix:
print("\t".join(map(str, line)))
A, B = read(options.filename)
A = scipy.matrix(A)
B = scipy.matrix(B)
C = A * B # easy and intuitive, isn't it?
printMatrix(C)
real 1m35.795s
user 1m33.438s
sys 0m0.488s
Conclusion for Python ¶
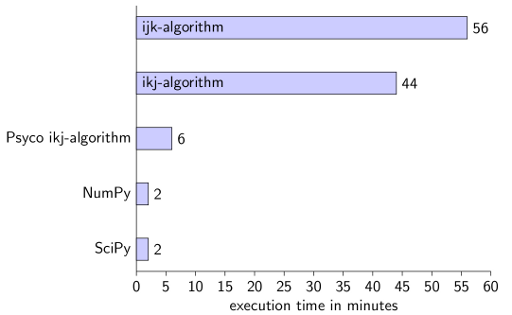
Using NumPy is by far the easiest and fastest option. I've needed about five minutes for each of the non-library scripts and about 10 minutes for the NumPy/SciPy scripts.
By the way, it is useless to combine Psyco and NumPy. It gets a little bit faster (1 minute and 28 seconds), but this could also be a random effect. If you execute it many times, you will see that the execution time is never the same.
Java ¶
I am using this Java version:
$ java -version
java version "1.6.0_20"
OpenJDK Runtime Environment (IcedTea6 1.9.13) (6b20-1.9.13-0ubuntu1~10.04.1)
OpenJDK Server VM (build 19.0-b09, mixed mode)
ijk-algorithm ¶
import java.io.BufferedReader;
import java.io.FileReader;
import java.io.IOException;
import java.util.LinkedList;
import java.util.List;
import java.util.ArrayList;
public class Shell {
static List<ArrayList<ArrayList<Integer>>> read(String filename) {
ArrayList<ArrayList<Integer>> A = new ArrayList<ArrayList<Integer>>();
ArrayList<ArrayList<Integer>> B = new ArrayList<ArrayList<Integer>>();
String thisLine;
try {
BufferedReader br = new BufferedReader(
new FileReader(filename));
// Begin reading A
while ((thisLine = br.readLine()) != null) {
if (thisLine.trim().equals("")) {
break;
} else {
ArrayList<Integer> line = new ArrayList<Integer>();
String[] lineArray = thisLine.split("\t");
for (String number : lineArray) {
line.add(Integer.parseInt(number));
}
A.add(line);
}
}
// Begin reading B
while ((thisLine = br.readLine()) != null) {
ArrayList<Integer> line = new ArrayList<Integer>();
String[] lineArray = thisLine.split("\t");
for (String number : lineArray) {
line.add(Integer.parseInt(number));
}
B.add(line);
}
br.close();
} catch (IOException e) {
System.err.println("Error: " + e);
}
List<ArrayList<ArrayList<Integer>>> res = new LinkedList<ArrayList<ArrayList<Integer>>>();
res.add(A);
res.add(B);
return res;
}
static int[][] ijkAlgorithm(ArrayList<ArrayList<Integer>> A,
ArrayList<ArrayList<Integer>> B) {
int n = A.size();
// initialise C
int[][] C = new int[n][n];
for (int i = 0; i < n; i++) {
for (int j = 0; j < n; j++) {
for (int k = 0; k < n; k++) {
C[i][j] += A.get(i).get(k) * B.get(k).get(j);
}
}
}
return C;
}
static void printMatrix(int[][] matrix) {
for (int[] line : matrix) {
int i = 0;
StringBuilder sb = new StringBuilder(matrix.length);
for (int number : line) {
if (i != 0) {
sb.append("\t");
} else {
i++;
}
sb.append(number);
}
System.out.println(sb.toString());
}
}
public static void main(String[] args) {
String filename;
if (args.length < 2) {
filename = "2000.in";
} else {
filename = args[1];
}
List<ArrayList<ArrayList<Integer>>> matrices = read(filename);
ArrayList<ArrayList<Integer>> A = matrices.get(0);
ArrayList<ArrayList<Integer>> B = matrices.get(1);
int[][] C = ijkAlgorithm(A, B);
printMatrix(C);
}
}
real 27m21.295s
user 26m53.877s
sys 0m4.368s
Note: Java is not C++! If you use Vector instead of ArrayList, you get these results:
real 82m26.754s
user 80m42.003s
sys 0m24.598s
One reason might be that Vector is synchronized.
ikj-algoirthm ¶
I've only switched line 60 and line 61.
real 2m9.478s
user 1m26.369s
sys 0m39.162s
Library: JAMA ¶
I've searched in Google for "java matrix multiplication". The first 10 results were only implementations of the ijk-algorithm. Although the ijk-algorithm is very easy, most of the results were only questions where people tried to implement it.
After some search (20 minutes minimum) I've found JAMA. They also have a documentation. You might need to install this for the following code:
sudo apt-get install libjama-*
import java.io.BufferedReader;
import java.io.FileReader;
import java.io.IOException;
import java.util.ArrayList;
import java.util.LinkedList;
import java.util.List;
import Jama.Matrix;
public class Shell {
static List<ArrayList<ArrayList<Double>>> read(String filename) {
ArrayList<ArrayList<Double>> A = new ArrayList<ArrayList<Double>>();
ArrayList<ArrayList<Double>> B = new ArrayList<ArrayList<Double>>();
String thisLine;
try {
BufferedReader br = new BufferedReader(new FileReader(filename));
// Begin reading A
while ((thisLine = br.readLine()) != null) {
if (thisLine.trim().equals("")) {
break;
} else {
ArrayList<Double> line = new ArrayList<Double>();
String[] lineArray = thisLine.split("\t");
for (String number : lineArray) {
line.add((double) Integer.parseInt(number));
}
A.add(line);
}
}
// Begin reading B
while ((thisLine = br.readLine()) != null) {
ArrayList<Double> line = new ArrayList<Double>();
String[] lineArray = thisLine.split("\t");
for (String number : lineArray) {
line.add((double) Integer.parseInt(number));
}
B.add(line);
}
} catch (IOException e) {
System.err.println("Error: " + e);
}
List<ArrayList<ArrayList<Double>>> res = new LinkedList<ArrayList<ArrayList<Double>>>();
res.add(A);
res.add(B);
return res;
}
static int[][] ijkAlgorithm(ArrayList<ArrayList<Integer>> A,
ArrayList<ArrayList<Integer>> B) {
int n = A.size();
// initialise C
int[][] C = new int[n][n];
for (int i = 0; i < n; i++) {
for (int j = 0; j < n; j++) {
for (int k = 0; k < n; k++) {
C[i][j] += A.get(i).get(k) * B.get(k).get(j);
}
}
}
return C;
}
static void printMatrix(Matrix matrix, int n) {
for (int i = 0; i < n; i++) {
for (int j = 0; j < n; j++) {
if (j != 0) {
System.out.print("\t");
}
System.out.printf("%.0f", matrix.get(i, j));
}
System.out.println("");
}
}
public static void main(String[] args) {
String filename;
if (args.length < 2) {
filename = "2000.in";
} else {
filename = args[1];
}
List<ArrayList<ArrayList<Double>>> matrices = read(filename);
ArrayList<ArrayList<Double>> A = matrices.get(0);
ArrayList<ArrayList<Double>> B = matrices.get(1);
int n = A.size();
double[][] Aarray = new double[n][n];
double[][] Barray = new double[n][n];
for (int i = 0; i < n; i++) {
for (int j = 0; j < n; j++) {
Aarray[i][j] = A.get(i).get(j);
Barray[i][j] = B.get(i).get(j);
}
}
Matrix AM = new Matrix(Aarray);
Matrix BM = new Matrix(Aarray);
Matrix CM = AM.times(BM);
printMatrix(CM, n);
}
}
real 1m36.506s
user 0m51.367s
sys 0m45.043s
It took me about two hours to get it work. I had to add the JAMA-JAR to eclipse, export my project as a JAR and run it with
$ time java -jar jama-shell.jar -i ../2000.in > jama-result.out
I still have no idea how to compile it with bash only.
Conclusion for Java ¶
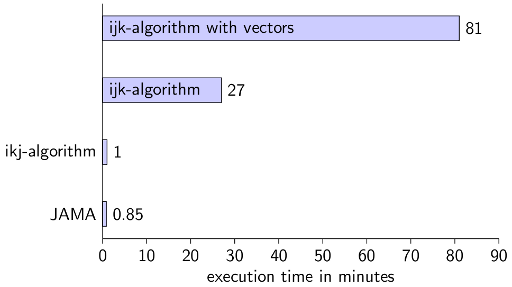
You should definitely know if some Java-datastructures are synchronised or not. And you should know how the computer / caches work.
C++ ¶
I have gcc 4.4.3 and compiled everything with these options:
$ g++ -std=c++98 -Wall -O3 -g myScript.cpp -o $(PROBLEM).out -pedantic
ijk-algorithm ¶
#include <sstream>
#include <string>
#include <fstream>
#include <iostream>
#include <vector>
using namespace std;
struct Result {
vector< vector<int> > A;
vector< vector<int> > B;
};
Result read(string filename) {
vector< vector<int> > A, B;
Result ab;
string line;
ifstream infile;
infile.open (filename.c_str());
int i = 0;
while (getline(infile, line) && !line.empty()) {
istringstream iss(line);
A.resize(A.size() + 1);
int a, j = 0;
while (iss >> a) {
A[i].push_back(a);
j++;
}
i++;
}
i = 0;
while (getline(infile, line)) {
istringstream iss(line);
B.resize(B.size() + 1);
int a;
int j = 0;
while (iss >> a) {
B[i].push_back(a);
j++;
}
i++;
}
infile.close();
ab.A = A;
ab.B = B;
return ab;
}
vector< vector<int> > ijkalgorithm(vector< vector<int> > A,
vector< vector<int> > B) {
int n = A.size();
// initialise C with 0s
vector<int> tmp(n, 0);
vector< vector<int> > C(n, tmp);
for (int i = 0; i < n; i++) {
for (int j = 0; j < n; j++) {
for (int k = 0; k < n; k++) {
C[i][j] += A[i][k] * B[k][j];
}
}
}
return C;
}
void printMatrix(vector< vector<int> > matrix) {
vector< vector<int> >::iterator it;
vector<int>::iterator inner;
for (it=matrix.begin(); it != matrix.end(); it++) {
for (inner = it->begin(); inner != it->end(); inner++) {
cout << *inner;
if(inner+1 != it->end()) {
cout << "\t";
}
}
cout << endl;
}
}
int main (int argc, char* argv[]) {
string filename;
if (argc < 3) {
filename = "2000.in";
} else {
filename = argv[2];
}
Result result = read (filename);
vector< vector<int> > C = ijkalgorithm(result.A, result.B);
printMatrix(C);
return 0;
}
real 1m40.439s
user 1m38.642s
sys 0m0.280s
ikj-algorithm ¶
Again, I've only switched line 61 and 62.
real 0m15.172s
user 0m14.877s
sys 0m0.248s
Library: Boost ¶
If you want to compile these scripts, you might have to install the boost libraries first. On Ubuntu you can enter:
sudo apt-get install libboost-math*
#include <sstream>
#include <string>
#include <fstream>
#include <iostream>
#include <vector>
#include <boost/numeric/ublas/matrix.hpp>
#include <boost/numeric/ublas/io.hpp>
using namespace std;
struct Result {
boost::numeric::ublas::matrix<int> A;
boost::numeric::ublas::matrix<int> B;
};
int getMatrixSize(string filename) {
string line;
ifstream infile;
infile.open (filename.c_str());
getline(infile, line);
return count(line.begin(), line.end(), '\t') + 1;
}
void printMatrix(boost::numeric::ublas::matrix<int> matrix) {
for (unsigned int i=0; i < matrix.size1(); i++) {
for (unsigned int j=0; j < matrix.size2(); j++) {
cout << matrix(i, j);
if(j+1 != matrix.size2()) {
cout << "\t";
}
}
cout << endl;
}
}
Result read(string filename) {
Result ab;
string line;
ifstream infile;
infile.open (filename.c_str());
// get dimension
getline(infile, line);
int n = getMatrixSize(filename);
boost::numeric::ublas::matrix<int> A(n,n), B(n,n);
// process first line
istringstream iss(line);
int a, i = 0, j = 0;
while (iss >> a) {
A(i,j) = a;
j++;
}
i++;
while (getline(infile, line) && !line.empty()) {
istringstream iss(line);
j = 0;
while (iss >> a) {
A(i,j) = a;
j++;
}
i++;
}
i = 0;
while (getline(infile, line)) {
istringstream iss(line);
j = 0;
while (iss >> a) {
B(i,j) = a;
j++;
}
i++;
}
infile.close();
ab.A = A;
ab.B = B;
return ab;
}
int main (int argc, char* argv[]) {
string filename;
if (argc < 3) {
filename = "2000.in";
} else {
filename = argv[2];
}
Result result = read (filename);
boost::numeric::ublas::matrix<int> C;
C = boost::numeric::ublas::prod(result.A, result.B);
printMatrix(C);
return 0;
}
real 4m15.388s
user 4m10.272s
sys 0m0.588s
Library: Blitz ¶
This is a great example of useless library. I've installed the library:
$ sudo apt-get install libblitz*
Then I wanted to use it. Well, I have no clue how I could exactly use it! See my StackOverflow Question: Is a documentation of Blitz++ matrices available?
Conclusion for C++ ¶
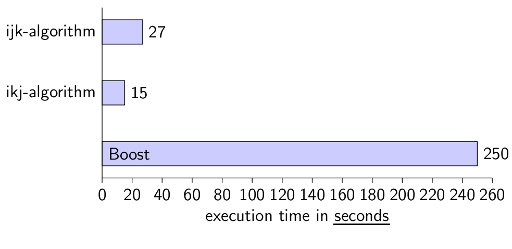
Again, it brings a performance boost if you know how your CPU works. I was very astonished, that the library Boost is slower (actually MUCH slower) than my simplest approach was.
Conclusion ¶
If I want to create a working piece of code in a minimum amount of time, I will always take Python. It has been very easy to solve this task with the given restrictions. But C++ is amazing when speed is important.
It was astonishingly difficult to find working code examples for this task for Java and C++. I was searching for libraries and found some, but the search results were not satisfying.
See also ¶
- Boost Matrix multiplication
- Rosetta Code: Matrix multiplication (Implementations in 63 programming languages!)
- Why is matrix multiplication faster with numpy than with ctypes in Python?
- Why is boosts matrix multiplication slower than mine?